
- Fecha de publicación
- maig 2022
- Tecnología
- Artículo
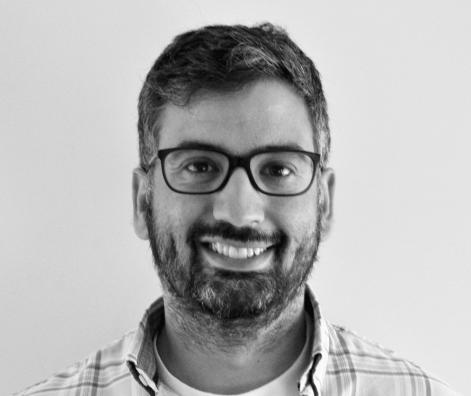
Profesor de ESIC. Responsable de Data Gobernance en Viewnext (grupo IBM).
Ha estado ligado durante los últimos 15 años a posiciones de Data Science, Data Managment, especializado en gestión de información, técnicas de geomarketing y estadísticas.
From this place, from here, from a structured article on a topic that is very much in vogue nowadays, I intend to elaborate and shed some light on what Deep Learning is, one of the most cutting-edge methodological frameworks in analytics.
To start such articles or sessions, I think it is always best to clarify the concept with a formal definition:
Deep learning is a set of machine learning algorithms that attempt to model high-level abstractions in data using computational architectures that support multiple and iterative nonlinear transformations of data expressed in matrix or tensor form.
It's a good way to avoid putting our foot in our mouth at the beginning of an article and to lay out the foundation, but do we really understand what we are talking about when we use the term deep learning? In the most basic and direct way, deep learning brings together a set of techniques that allow us to tackle problems that cannot be tackled with traditional techniques due to the complexity of the input data. This complexity is mainly due to the nature of the data to be processed, the unstructured data.
It is important to note that these techniques are not only oriented to the analysis of non-tabular data, such as images, videos, audio or text, but can also be applied to structured data when, for example, the volume of data is so large that it is not feasible with traditional techniques.
This is where it is up to the analyst or data scientist to decide how to approach a problem. As a general rule and as a maxim that facilitates the selection of the correct path, the principle of parsimony or Ockham's razor appears: "All things being equal, the simplest explanation is usually the most probable".
At this point, it is time to look at where it is used today, in which datasets:
Images.
It has always been possible to analyze pixel values found in a photograph or similar things, but deep learning can detect patterns and linkages between previously un-processable sets of pixels. With all this, predictive models can be derived.
This can be found in all kinds of photographic filters or in the estimation of the characteristics of a set of images, such as estimating the age of a person or the breed of a dog.
Videos.
Until now extracting the elements of a video, analyzing them and obtaining results from them was not easy, especially when you think of the time required to do so. Now, being able to do this "in real time" gives a new dimension to its use.
Both the detection of entities from a video (heat maps of a soccer player) and the generation of content (deepfake) are part of our everyday life.
Audio.
This includes not only the processing of audio inputs, but also the generation of content or speech. Once again, the speed of processing and the instantaneous availability of the audio output give new techniques a new dimension.
The standard in this area is virtual assistants, especially in "smart" speakers, where input audio is processed and output content is generated.
Text.
In this aspect, moving away from traditional word clouds or term counts, the new techniques push analytics towards context, towards linking terms in a document and even towards the creation of new texts.
The greatest exponent of this type of data is in natural language processing (NLP) and, taken to its most everyday use, it can be found in document management or in sentiment analysis (SSR).
I think it would be interesting to end with a couple of more specific mentions that allow us to narrow down this complex world a little more:
Deep learning ≠ neural networks.
Both terms are used in an analogous way and it is easy to commit this small error, since within the general framework of deep learning neural networks are the most used and the most developed in these years. Other techniques such as random forest can be found, but it is true that this analogy refers to the fact that neural network technology is the main bet, among others, of leading companies such as Google (www.tensorflow.org), which makes us start talking about a part as the whole.
Why do we talk about artificial intelligence when we mean deep learning?
Here we enter into more marketing terms or more advertising than academic terms. An intelligent speaker or car is always more marketable than a speaker or a car with deep learning models.
But the reality is that autonomous cars chain models that allow video recognition (front and rear cameras), image detection (signals), sensorisation (breakdowns) and all this processed in real time to facilitate the driver's decision making or even anticipate it automatically.
A smart speaker is able to recognize the different voice of regular users, transcribe the content of what they express, perform content searches and generate a response, either in the form of speech or output.
By way of summary, Alexa is not smart; it is technologically very advanced.
In conclusion, deep learning is complex, novel and opens up a whole world of possibilities, but we must always try to limit its scope, its definition and its reality so as not to inflate expectations that may end up puncturing or blur its real potential in erroneous terminologies or analogies.
También te puede interesar
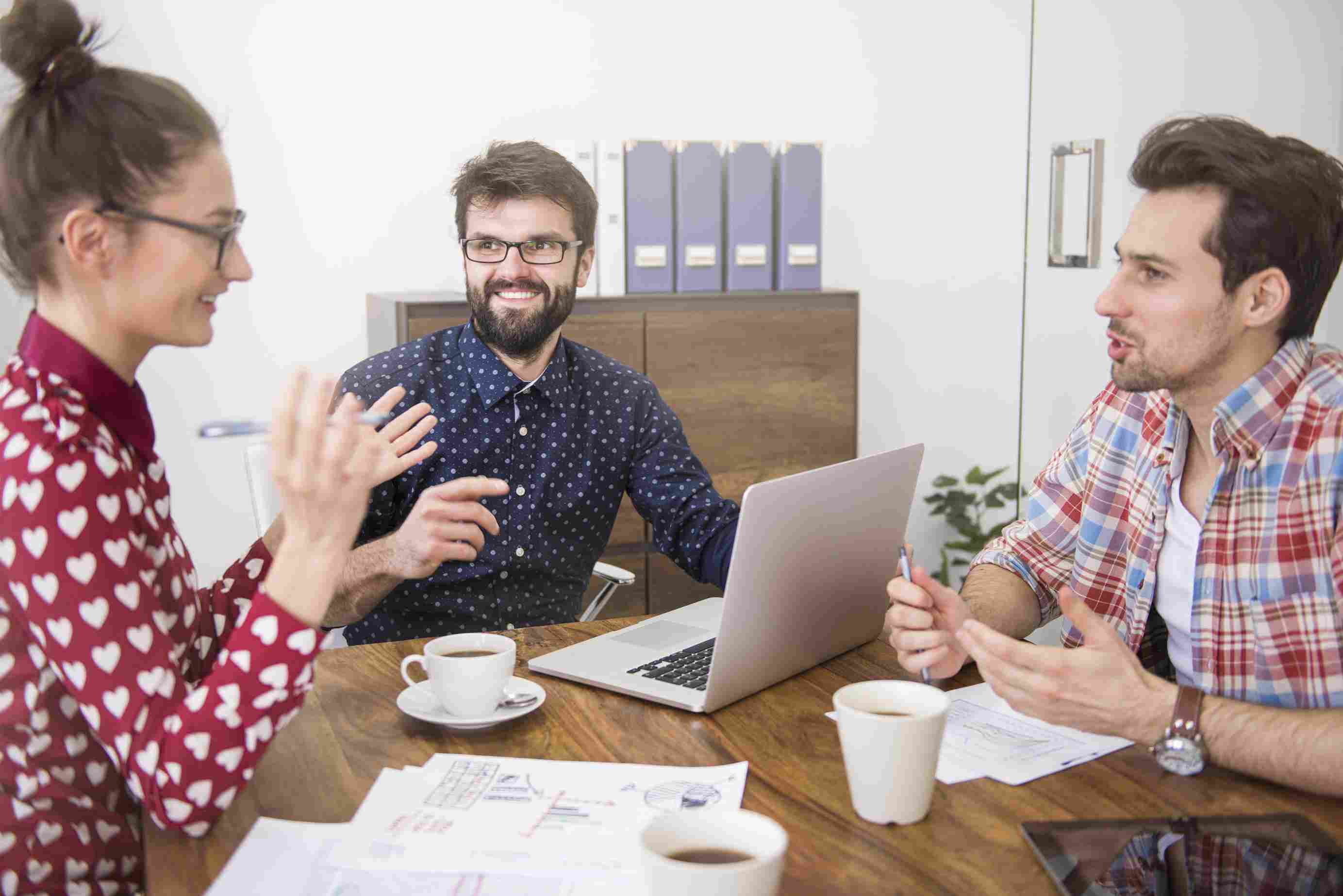
Team building: qué es y cómo podemos implementarlo en una empresa
Los empleados son la estructura de cualquier empresa. Sin ellos, el negocio no saldría adelante, motivo suficiente para tener departamentos encargados tan solo de buscar estrategias para que los empl...
- Publicado por _ESIC Business & Marketing School
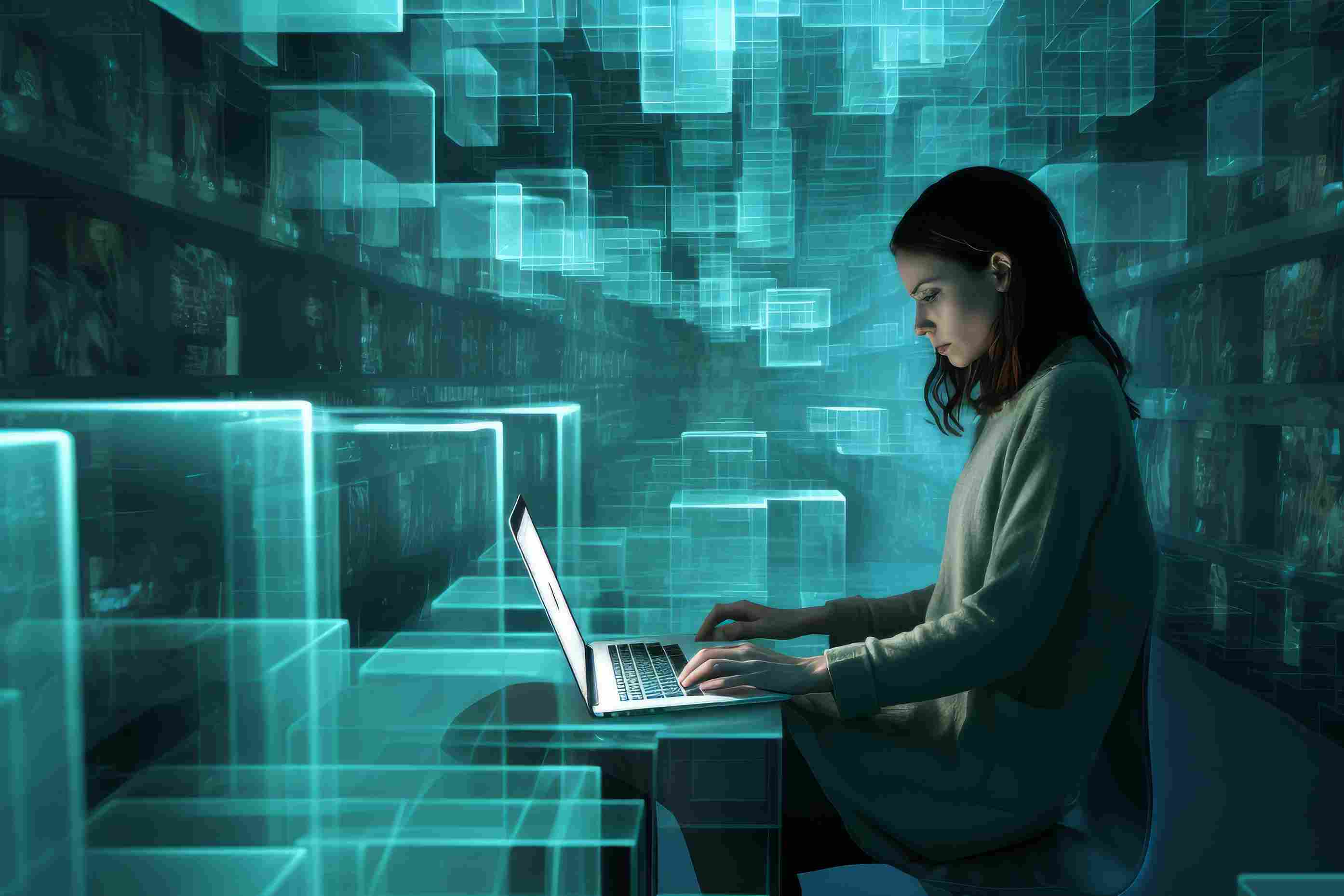
Qué es el modelo zero trust y cómo ayuda a mejorar la seguridad de tu empresa
La pandemia de covid-19 supuso un antes y un después en el funcionamiento de las compañías. La sociedad al completo se vio obligada a trabajar desde casa, lo que hizo que las empresas tuvieran que ...
- Publicado por _ESIC Business & Marketing School
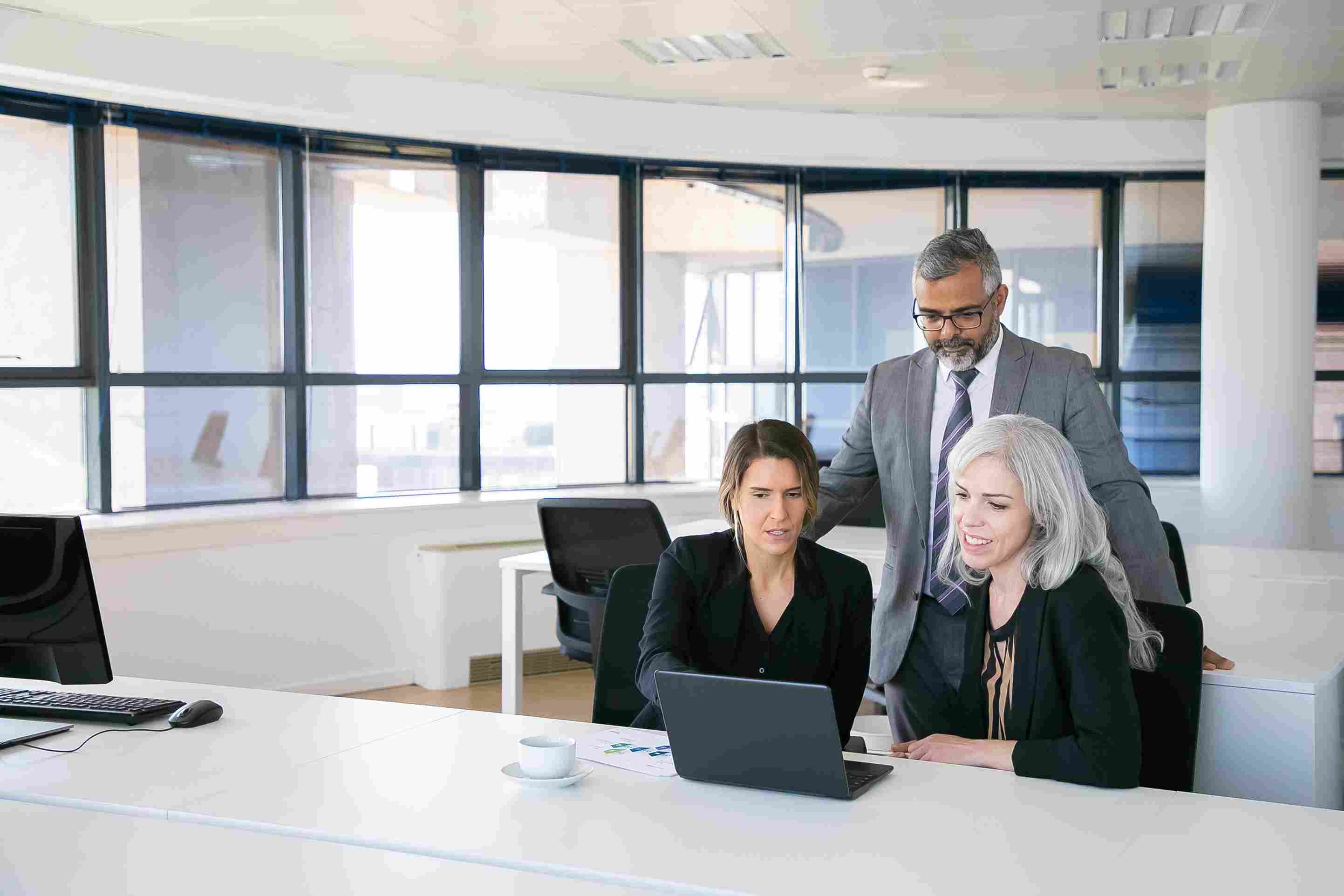
Dirección estratégica: qué es, para qué sirve y ejemplos
¿Vale todo el mundo para dirigir una compañía? Ser ambicioso y querer ascender en una empresa es, por supuesto, algo positivo y gratificante. Pero ¿qué ocurre cuando muchas personas llegan al pun...
- Publicado por _ESIC Business & Marketing School